High Dimensional Data Analysis With Low Dimensional Models

High dimensional data is becoming increasingly common in many fields, such as genomics, proteomics, and finance. This type of data can be difficult to analyze, as it can be difficult to visualize and understand the relationships between the different variables. However, there are a number of techniques that can be used to analyze high dimensional data, including low dimensional models.
Low dimensional models are mathematical models that can be used to represent high dimensional data in a lower dimensional space. This can make the data easier to visualize and understand, and it can also improve the accuracy of machine learning algorithms.
There are a number of different types of low dimensional models, including:
5 out of 5
Language | : | English |
File size | : | 25795 KB |
Print length | : | 440 pages |
Screen Reader | : | Supported |
- Principal component analysis (PCA): PCA is a linear transformation that can be used to reduce the dimensionality of data by identifying the principal components, which are the directions of greatest variance in the data.
- Linear discriminant analysis (LDA): LDA is a linear transformation that can be used to reduce the dimensionality of data by finding the directions that best separate different classes of data.
- Factor analysis: Factor analysis is a statistical technique that can be used to identify the underlying factors that explain the relationships between different variables.
- Manifold learning: Manifold learning is a technique that can be used to learn the low dimensional structure of data that is embedded in a high dimensional space.
The choice of which low dimensional model to use depends on the specific data set and the goals of the analysis.
Low dimensional models have a wide range of applications, including:
- Data visualization: Low dimensional models can be used to visualize high dimensional data in a way that is easier to understand. This can help researchers to identify patterns and relationships in the data that would be difficult to see with the naked eye.
- Machine learning: Low dimensional models can be used to improve the accuracy of machine learning algorithms. By reducing the dimensionality of the data, it is possible to make the data more manageable for machine learning algorithms and to reduce the risk of overfitting.
- Dimensionality reduction: Low dimensional models can be used to reduce the dimensionality of data for a variety of purposes, such as storage, transmission, and analysis.
There are a number of challenges associated with high dimensional data analysis, including:
- The curse of dimensionality: The curse of dimensionality is a phenomenon that occurs when the number of variables in a data set increases. This can make it difficult to visualize and understand the data, and it can also lead to overfitting in machine learning algorithms.
- Noise and outliers: High dimensional data is often noisy and contains outliers. This can make it difficult to identify patterns and relationships in the data.
- Computational complexity: Analyzing high dimensional data can be computationally expensive. This can make it difficult to use traditional statistical methods, and it can also make it difficult to scale up to large data sets.
High dimensional data analysis is a challenging but important problem. Low dimensional models can be used to address many of the challenges associated with high dimensional data analysis, and they have a wide range of applications.
As the amount of high dimensional data continues to grow, it is likely that low dimensional models will become increasingly important. These models can help us to better understand the world around us and to make better decisions.
5 out of 5
Language | : | English |
File size | : | 25795 KB |
Print length | : | 440 pages |
Screen Reader | : | Supported |
Do you want to contribute by writing guest posts on this blog?
Please contact us and send us a resume of previous articles that you have written.
Book
Novel
Page
Chapter
Text
Story
Genre
Reader
E-book
Magazine
Newspaper
Sentence
Shelf
Foreword
Preface
Scroll
Codex
Classics
Biography
Autobiography
Reference
Encyclopedia
Thesaurus
Narrator
Character
Borrowing
Stacks
Archives
Periodicals
Study
Scholarly
Reserve
Academic
Journals
Reading Room
Interlibrary
Study Group
Storytelling
Reading List
Theory
Wayne D Cottrell
Bryanna Farris
Noah Berlatsky
Rachel Morgan
Cleo Peitsche
Devin Edmonds
Joe Minichino
Richard La Ruina
Jay Newman
Joshua Seigal
Graham Field
Sahra Wagenknecht
Charles River Editors
Philip F Rubio
Ann Garcia
George D Robson
John Robert Greene
John Scura
Tina Cassidy
David Solomons
Light bulbAdvertise smarter! Our strategic ad space ensures maximum exposure. Reserve your spot today!
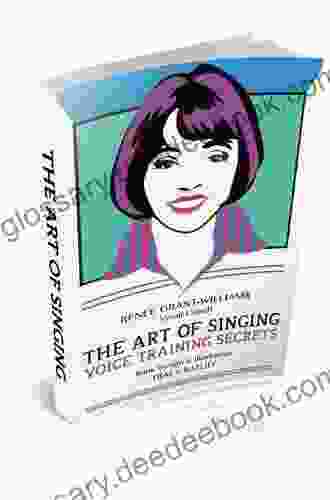

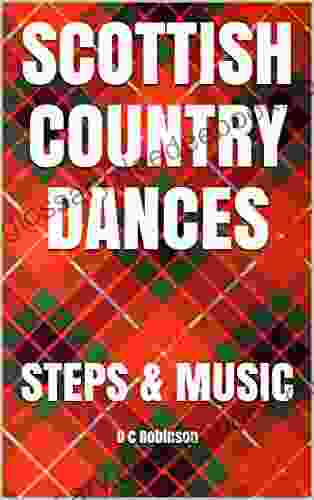

- Derek BellFollow ·15k
- Mark TwainFollow ·11.1k
- Emilio CoxFollow ·3.1k
- Ron BlairFollow ·6.6k
- George Bernard ShawFollow ·3.4k
- Houston PowellFollow ·6.6k
- Ethan MitchellFollow ·6.1k
- Al FosterFollow ·12.9k
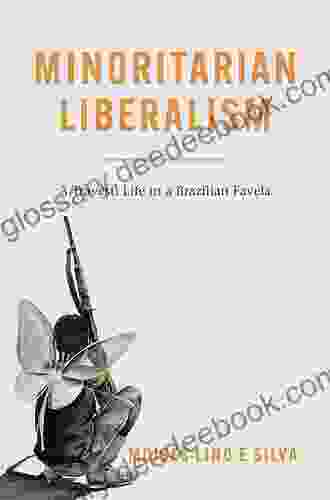

Travesti Life in the Favela: An Exploration of Identity,...
In the bustling...


Corruption and Development in South Korea and the...
Corruption is a major...


Gaslighting, Blame Shifting, and Consent in Marriage: A...
Gaslighting,...
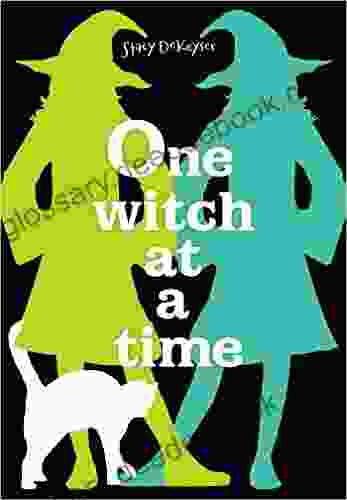

One Witch at a Time: Dive into the Enchanting World of...
Welcome to the Mystical Realm of...


Latino Mass Mobilization: Immigration, Racialization, and...
Latino mass...
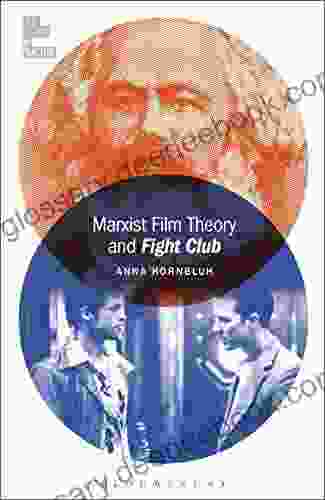

Marxist Film Theory and Fight Club: A Long-Tail...
Marxist film theory,...
5 out of 5
Language | : | English |
File size | : | 25795 KB |
Print length | : | 440 pages |
Screen Reader | : | Supported |